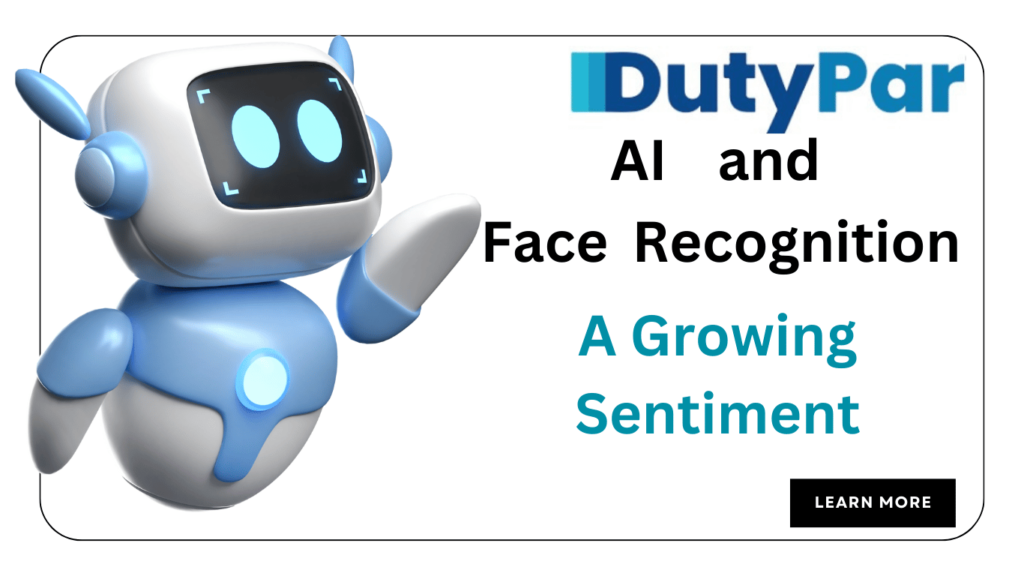
Table of contents
Introduction
A lot of industries are being changed today with the use of Artificial Intelligence (AI) in healthcare and finance, but much is being used in surveillance and security. Amongst the most popular methods in an AI application is the face recognition method. This is where the individual’s identity is verified based on facial features. This technology promises security, simplification of procedures, and customer experience. However, there is one critical concern regarding its possible discrimination against face recognition systems. For the most part, these concerns arise from biases within AI algorithms in relation to possible implications on privacy, civil rights, and social equality.
What is AI-based Face Recognition?
Face recognition through artificial intelligence scans images or videos utilizing machine learning algorithms, detecting and identifying a human face. The algorithms work on the basis of neural networks that have been trained on enormous amounts of datasets, distinguishing the unique features of faces such as space between the eyes, the shape of the nose, or jawline. Then it can match any image given with one that might be found in the database, or verify the identity of a particular person.
Potential Discrimination of Face Recognition
While it creates new opportunities, AI is not fault-proof. The main issue with the face recognition system will be that it discriminates against individuals. Bias could lead to people being wronged and injured in law enforcement, employment, and public services because AI systems suffer from training bias, inherent bias by developers, and technical limitations.
- Bias in Training Data
- The quality of AI-based face recognition essentially depends upon the diversity and quality of the training data. If an AI system is trained on a dataset that mainly contains people belonging to a particular racial background, gender, or age bracket, then identification of others outside that bracket would be biased. For instance, if an AI is mostly trained on light-skinned people, then it might not be able to correctly identify dark-skinned people and result in false positives, through inaccurate identification.
- Algorithmic Bias
Algorithmic bias refers to these unconscious discriminations that surface when developing AI. Sometimes, biases will creep into the system because of choices programmers make when coding, tuning, or optimizing algorithms. Even small biases build up into unfairly skewed outcomes that ripple when scaled up thousands or millions of times. - Bias in Policing
Probably the most controversial use of this face recognition technology has been in policing. AI-based face recognition has been abused by police forces all over the world to monitor and detect suspects. Despite the findings that these systems may have bias based on race and gender results in erroneous arrests and harassment of certain populations. This has further brought to the debate privacy, civil rights, and the proper usage of AI in policing. - Gender and Age Bias
Based on gender and age bias, AI-based face recognition systems also show biases. Many studies have come across the fact that a few systems are less accurate for women compared to men and for senior citizens compared to youngsters. This biased gender and age, therefore, causes false identifications and raises ethical concerns in most areas where precision identification is inevitable.
How IndoAI and DutyPar Tackle the AI Bias
While face recognition technology has the potential to discriminate against individuals, companies like IndoAI and DutyPar are increasing efforts to decrease bias in AI systems. They ascribe to the fiduciary of openness, diversity in datasets, and development of ethical AI to steer towards a more equitable and usable AI ecosystem.
- Diversifying Dataset
IndoAI has been working hard on diversifying the datasets which AI algorithms are trained upon. IndoAI aggregates and curates images from diverse demographics to reduce bias which arises due to skewed training data. IndoAI focuses on a very wide range of ethnicities, age, and gender which goes into forming the overall context of an inclusive AI. - Transparency in AI Development
As expected, this problem of potential discrimination calls for transparency when dealing with face recognition. Companies like DutyPar emphasize that they should be transparent in how their AI models are trained, what data is used, and what measures are there to avoid bias. This will engender trust among users and allows audits of fairness by independent parties. - Bias Testing and Correction
IndoAI tests its AI-based face recognition solutions for any potential biases even before deployment. Realistic-case scenarios help measure the functioning of the AI system across many segments in the population. For correct measures, they fine-tune the algorithm and retrain with diverse data. This ensures continuous evolution of AI systems into more accurate ones. - Ethical Standards in AI
DutyPar upholds ethics concerning AI. They have set strict guidelines such that the AI systems they come up with do not discriminate. Their ethical standards entail banning biased data from being used, constant monitoring for biases in AI technology, and responsible deployment of this particular technology into sensitive sectors such as law enforcements and recruitment.
Real-Life Examples of Discrimination in Face Recognition
After all the efforts of companies like IndoAI and DutyPar, there is still a very strong potential for discriminatory activity in face recognition technology. Let’s now see some examples depicting the risks: - The Case of Amazon Rekognition
Amazon’s face recognition system, Rekognition, which in 2018 wrongfully identified members of Congress as people who had committed crimes; and the system showed a higher error rate for people of color, thus opening the pot to questions about biases in the AI algorithm. This was a critical incident of the problems that biased AI can bring into the very sensitive areas of policing.
The Gender Shades Project
Joy Buolamwini led on Gender Shades, the project that researched the performance of popular face recognition systems. She discovered that these systems made more mistakes in women and darker-skinned people. They established that there was a necessity for the proper training data and diverse datasets in AI-based face recognition to avoid biased results. - Bias in Hiring Systems
Other organizations have implemented facial recognition with the help of artificial intelligence to filter out job applicants by studying their facial features that can determine if they are a probable success or not. It may encourage biases in the result if data for training AI algorithms are not unbiased. Therefore, candidates with certain backgrounds face unfair disadvantages in the recruitment process.
Mitigating the Possible Discrimination of Face Recognition
In order to prevent the possibility of having face recognition discriminate against an individual based on his or her features, the following aspects of ethical concerns need to be addressed include: - Implementation of Regulations
The governments and regulatory bodies have to make proper protocols and standards for the use of face recognition technology. The laws should require it to be fair, accurate, and transparent in AI algorithms. And other data protection laws can prevent the misuse of face recognition in highly sensitive areas like law enforcement or hiring. - Public Awareness and Debate
The public needs much awareness and education about the potential for discrimination by face recognition systems. Through open debates and discussions, citizens can be made aware of how AI works, the existence of biases, and ways to improve fairness. This public dialogue can in turn propel policy and technology changes, including company pressures on the development of ethically sound AI. - Independent Audits and Accountability
Independent audits may be a crucial step to ensure that AI systems are fair and non-discriminatory. Companies should call upon to address biases in their algorithms and conduct regular audits themselves. These audits would go through the accuracy of AI systems across different demographics and make suggestions on potential areas for discriminations. - Ethical AI Design
Designing fair AI systems is important in preventing the probable discrimination that those systems may exert. Among these are diverse training data sets, preventing biased data collections, testing for unintended effects, and most importantly, developers of such AI have to adhere to some level of ethics. By integrating ethical considerations into the design phase, companies can create more fair AI from the get-go.
The Future of AI and Face Recognition
The reduction of existing discrimination and giving way to better future prospects is in the hands of AI-based face recognition technology. Companies like IndoAI and DutyPar lead by the benchmark of transparency, diversity, and high ethical standards. However, much collective effort from the developers of AI, policymakers, and members of the public will make a technology equal for all.
Conclusion
The serious consideration of discrimination based on facial features through the use of AI and facial recognition technology is something that needs to get ready. Our use of AI in our daily lives must then come with a consideration for ethical implications; it cannot perpetuate any already existing biases nor generate new ones. Companies such as IndoAI and DutyPar are spearheading efforts to build more equitable and transparent AI systems.. All players, from AI developers to policymakers, are responsible for ensuring that technology is offered equally and justly to everyone.