Machine Learning and Attendance Tracking: What You Need to Know
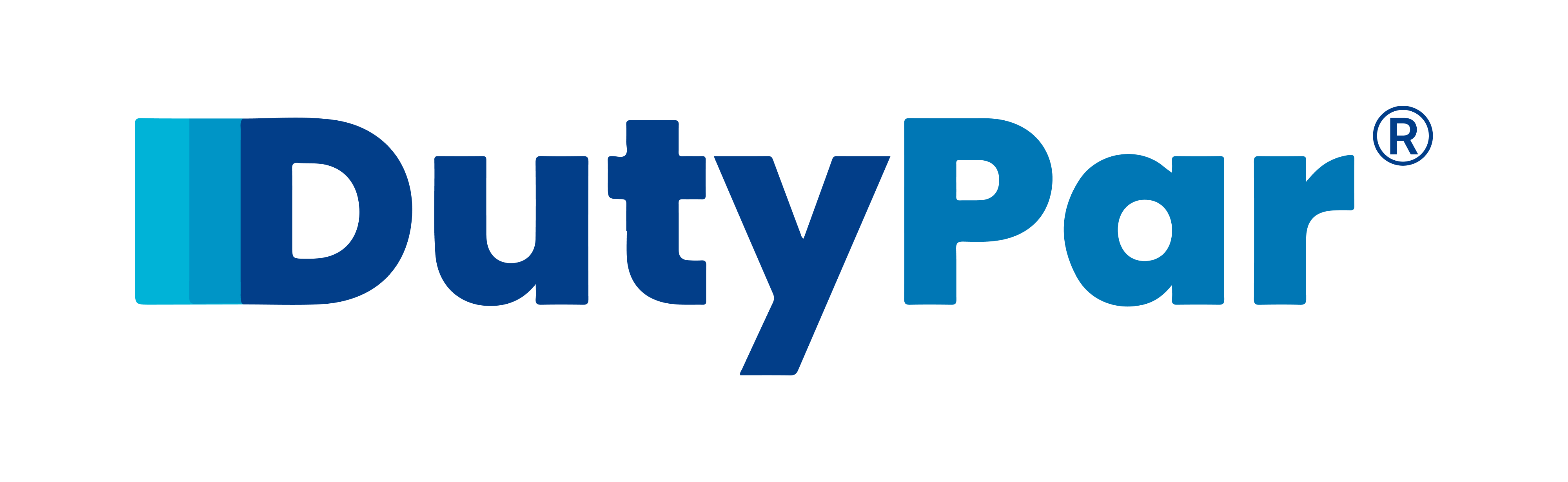
In today’s world, organizations are adopting innovative technologies to streamline their operations and improve efficiency. One such technology that has gained immense popularity is machine learning. Machine learning has revolutionized attendance tracking, making it easier and more accurate than ever before. In this blog post, we will explore what Machine Intelligence is and how it is being used for attendance tracking. We will also discuss the benefits of using machine learning for attendance tracking and what you need to know before implementing it.
What is Machine Learning?
Machine learning is a subset of artificial intelligence that involves training machines to learn from data and improve performance without being explicitly programmed. In other words, it is a process where machines can learn from the data they are given and make predictions or decisions based on that data. Machine learning algorithms can identify patterns, make predictions, and provide insights that can be used to optimize various processes.
How Machine Intelligence is Being Used for Attendance Tracking
Attendance tracking is a critical process for any organization, big or small. Traditionally, attendance tracking has been done manually, which can be time-consuming and error-prone. With machine learning, attendance tracking can be automated, making the process more efficient and accurate.
One way Machine Intelligence is being used for attendance tracking is through facial recognition technology.
Facial recognition technology uses algorithms to recognize and identify individual faces in a crowd. With the help of machine learning, attendance tracking systems can identify and record employees‘ faces as they enter and exit the workplace, eliminating the need for manual attendance tracking.
Another way Machine Intelligence is being used for attendance tracking is through predictive analytics.
Predictive analytics uses machine learning algorithms to analyze historical attendance data to predict future attendance patterns. This can be useful for organizations to plan ahead and adjust their workforce accordingly.
Benefits of Using Machine Learning for Attendance Tracking

There are several benefits of using machine learning for attendance tracking:
• Efficiency: Machine Intelligence automates the attendance tracking process, saving time and reducing the need for manual labor.
• Accuracy: Machine Intelligence algorithms can identify and record attendance data with a high degree of accuracy, reducing the risk of errors.
• Cost-effective: Automated attendance tracking can save organizations money by eliminating the need for additional personnel.
• Predictive analytics: Machine Intelligence algorithms can analyze historical attendance data and predict future attendance patterns, allowing organizations to plan ahead and adjust their workforce accordingly.
What You Need to Know Before Implementing Machine Learning for Attendance Tracking
Before implementing Machine Intelligence for attendance tracking, there are a few things you need to consider:
Accuracy: While Machine Intelligence algorithms can be accurate, they are not perfect. It is essential to conduct regular checks and maintain data accuracy.
Implementation: Implementing Machine Intelligence for attendance tracking requires a significant initial investment in hardware and software.
Conclusion
Machine Intelligence has revolutionized attendance tracking, making it easier and more accurate than ever before. Through facial recognition technology and predictive analytics, organizations can automate attendance tracking, save time and reduce the risk of errors. However, before implementing Machine Intelligence for attendance tracking, organizations need to consider accuracy and implementation costs. With proper planning and consideration, machine learning can be a game-changer for attendance tracking, improving efficiency and optimizing workforce management.