This blog explains the key differences between Natural Language Processing (NLP) and Machine Learning (ML), highlighting their distinct focuses, applications, and benefits, while also emphasizing how they complement each other in various industries. Understanding these differences helps businesses choose the right technology for specific applications.
Table of Contents
Introduction
Natural Language Processing (NLP) and Machine Learning (ML) are two popular technologies in the field of data science. Both of these technologies are widely used in industries such as healthcare, finance and marketing. These are not two completely different technologies but one is a use-case application of the other. However, there can be significant differences between NLP and ML in terms of application. Natural Language Processing (NLP) uses Machine learning (ML) models to analyze text and language. Natural Language Processing’s main goal is to teach computers to understand what is said in both spoken and written language.An NLP set of rules is put into action each time you dictate something into your iPhone or Android device, which then converts it into text.
In this article, we will explore the differences between NLP and ML, their applications, and the benefits they offer.
What Is Natural Language Processing?
You might hear phrases like what is NLP, what is NLP in AI, how does NLP work, what is nlp in machine learning, etc on a regular basis along with some complicated explanation for it, but simply put, Natural Language Processing (NLP) is a technology that enables computers to understand human language. It is a subfield of computer science, artificial intelligence, and linguistics that focuses on the interaction between human language and computers. NLP involves the use of algorithms, statistical models, and linguistic rules to analyze, interpret, and generate human language. The next question that comes to mind is what are the natural language processing steps. The chart below shows these steps while giving us a brief overview of the NLP process.
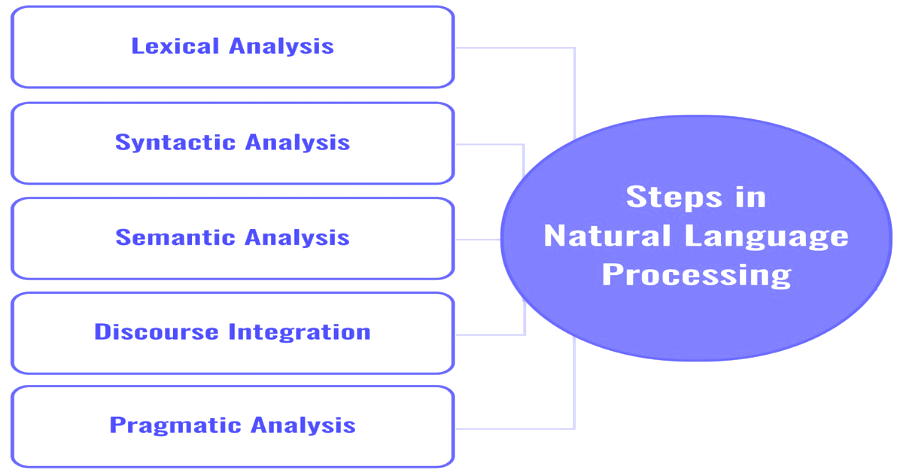
Now that we know the NLP process, one might wonder what is the field of natural language processing. Some of the most common fields of application include chatbots, virtual assistants, voice recognition systems, sentiment analysis, text classification, and machine translation.
Natural Language Processing (NLP) Applications
NLP applications across different industries include:
• Sentiment Analysis: The question “What is sentiment analysis?” can have different answers under different contexts but in our case Sentiment Analysis using machine learning is the process of analyzing the emotions, opinions, and attitudes expressed in text data. NLP algorithms can be used to identify and extract sentiments from large volumes of text data, such as social media posts, customer reviews, and feedback forms.
• Chatbots and Virtual Assistants: NLP is widely used in chatbots and virtual assistants to enable human-like interactions between users and machines. NLP algorithms can analyze user input and generate appropriate responses in natural language.
• Machine Translation: If like the rest of us you live on earth, chances are that you might have overheard people discussing questions like what is Machine Translation, what is Machine Translation in NLP, is it going to make multilingual skills useless, is Machine Translation in Artificial Intelligence. To answer it in simple terms Machine Translation is yet another miracle of Natural Language Processing. NLP is used in machine translation to translate text from one language to another. NLP algorithms can identify the structure of sentences in different languages and generate accurate translations.
• Text Classification: NLP is used in text classification to classify text data into different categories. This can be useful in applications such as email spam filtering, content categorization, and sentiment analysis. It also comes with its set of problems like claims that deep learning is not suitable for text analysis or questions like can we consider sentiment classification as a text classification problem.
What Is Machine Learning (ML)?
Machine Learning (ML) definition, simply put, can be a subset of artificial intelligence that can learn from data that is fed and get better with experience.
ML algorithms can identify patterns, make predictions, and improve their performance over time without being explicitly programmed. The Machine Learning process has applications in image recognition, speech recognition, recommendation systems, and predictive analytics.
Machine Learning Applications
ML has been touted as a major paradigm shift in the way industries operate. So what are the applications of machine learning that are so hyped these days. Some of the popular ML applications across different industries include:
• Image Recognition: ML algorithms and in particular a convolutional neural network is mainly used for image recognition; it can recognize objects, faces, and patterns in images. This can be useful in applications such as self-driving cars, security systems, and medical diagnosis.
• Speech Recognition: ML algorithms like recurrent networks work best for speech recognition. It can be used to recognize and transcribe human speech. This can be useful in applications such as virtual assistants, speech-to-text systems, speech emotion recognition and language translation.
• Recommendation Systems: ML algorithms can be used to make personalized recommendations based on user behavior and preferences. Such recommender systems can be useful in applications such as e-commerce, media, and entertainment. Content based recommendation system like movie recommendation system and book recommendation system are quickly gaining popularity.
• Predictive Analytics: ML algorithms can be used to analyze historical data and make predictions about future events. This can be useful in applications such as finance, healthcare, and marketing. Predictive analytics examples include predicting future cash flow, staffing needs and even market trends for businesses and other interested parties.
Differences Between Natural Language Processing And Machine Learning
Focus: The primary focus of NLP is on language processing, while the primary focus of ML is on building algorithms that can learn from data.
Data: NLP algorithms require text data, while ML algorithms can work with a variety of data types, such as numerical, categorical, and image data.
Approach: NLP involves the use of linguistic rules, statistical models, and algorithms to analyze, interpret, and generate human language. ML involves the use of algorithms to learn patterns and relationships in data without being explicitly programmed.
Application: NLP is used in applications that involve human language, such as chatbots, virtual assistants, sentiment analysis, and machine translation. ML is used in a variety of applications such as image recognition, speech recognition, recommendation systems, and predictive analytics.
Benefits: NLP enables computers to understand human language, which can improve communication and customer service. ML enables the automation of tasks and the generation of insights from large volumes of data, which can lead to cost savings and increased efficiency.
Conclusion
In summary, NLP and ML are two slightly distinct technologies that are used in a variety of applications across different industries. While NLP focuses on language processing, ML focuses on building algorithms that can learn from data. Understanding the differences between NLP and ML can help businesses make the right decisions about which technology to use in a specific application. Ultimately, both NLP and ML offer significant benefits and can be used together to achieve even greater results.